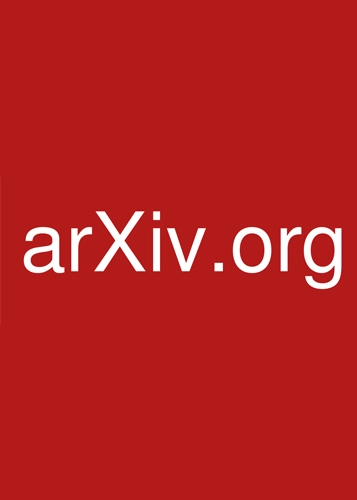
Unsupervised Learning of Depth, Optical Flow and Pose with Occlusion from 3D Geometry. (arXiv:2003.00766v3 [cs.CV] UPDATED)
Publisher URL: http://arxiv.org/abs/2003.00766
DOI: arXiv:2003.00766v3
Keeping up-to-date with research can feel impossible, with papers being published faster than you'll ever be able to read them. That's where Researcher comes in: we're simplifying discovery and making important discussions happen. With over 19,000 sources, including peer-reviewed journals, preprints, blogs, universities, podcasts and Live events across 10 research areas, you'll never miss what's important to you. It's like social media, but better. Oh, and we should mention - it's free.
Researcher displays publicly available abstracts and doesn’t host any full article content. If the content is open access, we will direct clicks from the abstracts to the publisher website and display the PDF copy on our platform. Clicks to view the full text will be directed to the publisher website, where only users with subscriptions or access through their institution are able to view the full article.